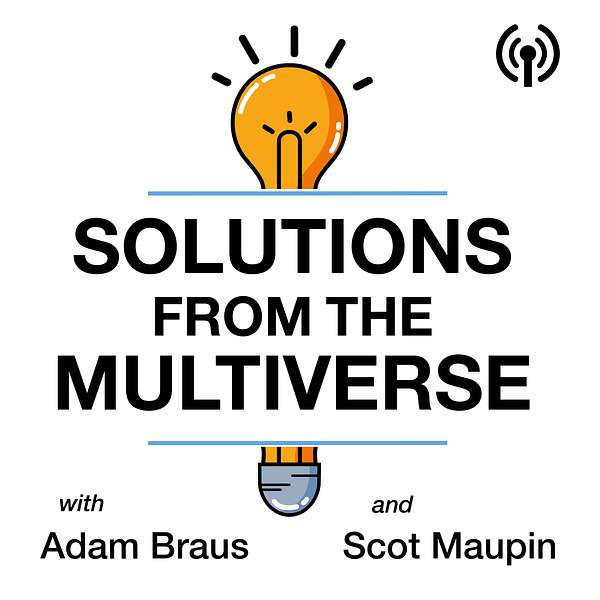
Solutions From The Multiverse
Hosts Adam Braus (@ajbraus) and Scot Maupin (@scotmaupin) meet up each week where Adam brings a new idea to help the world and Scot picks and prods at it with jokes and questions. The result is an informative and entertaining podcast that always gets you thinking.
Solutions From The Multiverse
Solving Ethical AI: Navigating the Ethical Challenges with Ben Byford | SFM E68
Imagine a world where artificial intelligence reigns supreme, making pivotal decisions that directly impact humanity. Sounds like a dystopian movie plot, right? Not in this thought-provoking episode with AI ethics expert Ben Byford. Ben unravels the complex web of AI ethics, shedding light on the importance of ethical considerations in AI deployment and consumption. How do we instil morality in a machine? Could AI ever be sentient? And what on earth is a "Jiminy Cricket" module for AI? Get ready for a deep dive into these pressing questions and more.
As we navigate the fascinating world of AI ethics, we take a pause to consider the human brain's vital role in our ethical decision-making processes. Taking a closer look at the amygdala, the brain's fear and aggression center, we explore its potential influence on AI behavior. The conversation then swerves into the ethical minefield of self-driving cars and the Moral Machine Net, a controversial project that grapples with life-or-death decisions.
Finally, we tackle the ethical challenges looming in AI development, dissecting the possible dangers of large language models and the need for stringent regulations. The impact of AI on humanity is a thrilling yet frightening prospect, and as our journey through AI ethics with Ben draws to a close, we leave you with one final thought: the future of AI ethics isn't just about machines—it's about us. So tune in, buckle up and prepare for a roller coaster ride into the world of AI ethics.
Connect with Ben Byford:
Website: https://benbyford.com/
Podcast: https://www.machine-ethics.net/
Social: https://www.linkedin.com/in/ben-byford/
Help these new solutions spread by ...
- Subscribing wherever you listen to podcasts
- Leaving a 5-star review
- Sharing your favorite solution with your friends and network (this makes a BIG difference)
Comments? Feedback? Questions? Solutions? Message us! We will do a mailbag episode.
Email: solutionsfromthemultiverse@gmail.com
Adam: @ajbraus - braus@hey.com
Scot: @scotmaupin
adambraus.com (Link to Adam's projects and books)
The Perfect Show (Scot's solo podcast)
The Numey (inflation-free currency)
Thanks to Jonah Burns for the SFM music.
Welcome everyone to Solutions from the Multiverse. Welcome, hello. I'm Adam Brouse, I'm Scott Moppen and we're met with a guest here, ben Biford.
Speaker 2:Hello.
Speaker 1:Hello, ben Welcome Ben.
Speaker 2:Hi, how are you guys doing?
Speaker 3:Good Doing well, a little panicked because I just woke up later than I wanted to.
Speaker 2:I know I'm here with a beer, like in the evening in the UK.
Speaker 3:I was just going to say I was working up, so I detect an accent. Where are you located, so?
Speaker 2:I'm in Bristol, in the sunny dreary, old England. It's actually raining outside the moment.
Speaker 1:Absolutely Perfect. That sounds great. You're either in England or you're just a raging alcoholic. You know beer on your Wheaties in the morning.
Speaker 2:I know when did you find me, man, right, he just picked me off the street, right, that's it.
Speaker 1:So we're talking to Ben. Ben is cool. He's a podcaster, hey, all right, we love that. We love that, that's right, very cool. And his podcast is called. It's called machineethicsnet, with a hyphen, if you want to go to the website Machineethicsnet. And and and I was on the podcast. Well, it's not live yet, but last just the other days, Ben recorded a pot in intro or interviewing me.
Speaker 2:About, yeah, ethics.
Speaker 1:It's going to be episode 82, I think, so that'll be out in like December, so check it out All right, so we so during that episode we kind of came like I kind of you know together but more me really. No offense, ben.
Speaker 3:Now that we're on Adams podcast.
Speaker 1:Well, I came up with a solution during it which actually it's in the book. It's already in the book. Really, it's kind of at the tail end of the AI chapter of my book on ethics. And so then I was like, oh well, maybe you should come on solutions and you can rip apart my bad idea and Ben was like sure, it's really stupid, so I'll be there to to rip it apart.
Speaker 3:I would welcome an I I would welcome an expert to help rip apart Adams bad ideas, because I've never qualified enough to do it.
Speaker 2:But I know, I know it must be done. There's something I know it must be done and yet I I need help. I think that's what good ideas are all about. Like, you want to have a good idea and you want to make it solid, right, so that that requires people to come and shoot you down and to like contribute, you know, in a positive way to that. That's why I'm, that's why I'm contributing here, that's why it's a new.
Speaker 3:Socratic math method right. The new Socratic method is. I have an idea and then everyone tells me how bad it is, instead of asking me questions.
Speaker 2:It's OK.
Speaker 1:Don't keep me in suspense.
Speaker 3:What is this?
Speaker 1:What is this masterful idea? Ok, so well. So we've established Ben is an expert AI ethics OK, yeah, Wait.
Speaker 3:what is that Hold on for someone who's not an expert in?
Speaker 2:what does that mean? So I run a podcast and I have done since 2016, all about AI and ethics and society and back. I don't know if you want this like preamble, but like back then there wasn't this idea of AI ethics and an AI ethicist and that people in humanities could really contribute in this area and it was kind of like it was sort of academic at the time and in business world it was non-existent. So I thought it was quite interesting Automated cars were coming in, all this stuff was happening, big data, iot and obviously vision and neural networks and stuff and I was like cool, like, but this is really interesting, but like, how is it actually going to, like you know, play out and affect us and what does that look like?
Speaker 2:So I start the podcast because I wanted to know from like cool people, more experienced people, people who are in this world, what they think and hopefully learn a load of stuff. So in that time, like I say, we're up to episode 18 now and we've been talking to all sorts of people and I've been using some of that knowledge and trying to do my own workshops and talk to and do talks and a device and I've done some consulting as well on AI ethics. But, like I say, it's weird and AI ethics is like a really recent thing and it wasn't a thing and now it is. So if you ask someone if they're an AI ethicist, they are allowed to say yes and it still feels like you're a charlatan, but it's fine.
Speaker 3:I was going to say AI ethics is making sure to program the robots to say please and thank you, right, is that? Do I have that? Yeah, correct, sure, what is it? What is it? What is that detail? I genuinely do not know. Is it just OK? Yeah, yeah.
Speaker 2:It's looking at and trying to help mitigate the ill effects of AI and automated algorithmic processes.
Speaker 3:So making sure we use AI in an quote unquote ethical way. Is that? Am I closer now? Yeah, it's like.
Speaker 2:It's like it's like full stack, let's say like full System. It's about the, the requirements of AI, is about the making of the AI and the data that they might use in the AI systems, like it's the deployment, it's the consumption, it's the whole chain of those things, like thinking about the whole thing and going OK, well, there might be issues here and here and here, and you're using people's data over here and where do you get that from? And having is the AI ethics term. In the AI ethics system, ethics term is more like cool. This person just knows a lot about ethics and also AI and is applying in that way to try and help mitigate ill outcomes, like all sorts of. There's all sorts of things that are that could go wrong.
Speaker 3:Do people like? Is there a general agreed on? Do people agree on what ethics like? What would be ethical or not Like? I feel like in human entity there's an argument where some people are like that would be ethical and other people like no, no, you know that's the struggle back and forth. Is that an already? Have we defined that for AI so far?
Speaker 2:I'm going to do that too, so I just so I just asked chat to.
Speaker 1:PT and chat to PT said AI ethics is a broad and evolving field with numerous important topics and sub topics here are key topics Bias and fairness, transparency and explainability, accountability and responsibility, privacy, security, consent, automation and unemployment, human oversight, bias in data and training, beneficence, non-malphysence, autonomous weapons and lethal AI. That was the sky.
Speaker 3:Let's say the mix is terminators.
Speaker 1:That means terminators, that's terminators.
Speaker 3:Yeah.
Speaker 1:Discrimination and AI and criminal justice, ai and healthcare, ethical considerations and research and ethical considerations and AI policy and regulation. So there you go, straight from the horse's bot mouth.
Speaker 3:Would an ethical AI refuse to like write a book report for you if you're trying to use it to like get through your homework a little faster? Would?
Speaker 2:it be like no, no, no.
Speaker 1:I think you might have hit across the.
Speaker 2:I think you might have hit across the issue that like, if someone's selling you a ethical AI and got air quotes here right, then they're probably you know that haven't. What does that mean? Like an ethical AI? So there's a problem there already. So I don't. I don't think there is. There is an ethical AI that people can tell you about at the moment, just for the people of AI.
Speaker 1:Just for people who aren't watching the video because there is no video but Ben is doing like really aggressive air quotes with his hands when he says ethical AI.
Speaker 1:So I'll just point out too, as a sort of compliment to Ben, that Ben is. Ben is an AI ethicist hipster. He was well into it before chat he PT launched, and there is this crazy spike. As soon as chat to be three launched, a bunch of like self promoting, like marketer tech bros were like, oh, I don't know how to code, so I'll call myself an AI ethicist, and they just put that into their like LinkedIn bio. They wrote a bunch of essays, probably with chat. They got a GPT and like put them on their blog and said, no, I'm not an AI ethicist, so Ben is actually the OG. Yeah, I think so.
Speaker 2:Yeah, I've got, I've got an amazing chat with someone who is like it's been in this area. So so what, as long as I have and it's going to be out the soon episode 81 and Alice, she really digs into all these people. She really hates it. She's like there's so much chatter and it's just all like rhetorical and horrible at the moment from all sorts of different people who don't know what they're talking about. That's not to say there is amazing people, but that is. It is become crowded right, sure, and amateurs.
Speaker 1:I mean, I'm all for amateur, so get in there, but but yeah, it is funny that you get this kind of whatever you want to call it, you know Okay so I think I understand the playing field.
Speaker 3:What's, what's the new player going to have us run at him? What are you doing?
Speaker 1:Okay, so this is the idea. So the idea is what I call a AI amygdala and art of computational amygdala, and this goes back to somewhat I don't know if exactly verifiable, but it's a hypothesis that I develop in my book about ethics, my about misrecording ism the least avoidable misery theory, the theory that what's ethical is what leads to the least avoidable misery, and the idea that I know what an amygdala is obviously because it's the name of a Batman villain and I read comic books as a kid.
Speaker 3:So, I understand this is the name of a super villain who, like gets real mad kind of you know his DC's Hulk guy gets real mad, gets kind of dumb and and just kind of gets aggressive Is. Is that accurate to what the amygdala is supposed?
Speaker 2:to do? Oh man, I should check that my Batman man amygdala.
Speaker 3:Batman villain named amygdala. Not a great costume design. I think he's just got like shredded T shirt normally, oh my God, it looks like he's bright red.
Speaker 1:He's red. Oh, he turns red now. Okay, he's totally red.
Speaker 3:He was just flesh colored when I was reading back then in the day. God damn.
Speaker 1:But that's the spot.
Speaker 3:That's the thing in your brain, right? The little tiny thing in your brain.
Speaker 1:There's two of them. It's an almond shaped organ that's about roughly above, right above in your ears and about an inch in on both sides. Okay, it's kind of on the temporal lobe, the side lobe of your brain.
Speaker 3:But more importantly, it's the location of fear mostly.
Speaker 1:Response to fear or concern, but also there's a kind of there's other kinds of tied into it with like aggression makes sense and then villain would get kind of aggressive because you know you can have sort of fear response like fight or flight. It's all kind of mediated by the amygdala, is like the sensor that senses whether a situation is distressful or not, and it can have effects on all kinds of emotional.
Speaker 3:There's already a Batman villain who deals with fear. Okay, which is the scarecrow?
Speaker 1:of course, big to love. Oh sorry, no, the scarecrow.
Speaker 3:Yeah, so they had. They had to go for the other side.
Speaker 1:They originally were gonna call him the hypothalamus.
Speaker 3:This is a Batman villain podcast, right when we just talk about different Batman villains. So there's I. There's time to the right Riddler the Riddler, the penguin, uh-huh, catwoman.
Speaker 1:Sure, of course, catwoman, although she's not really a villain, is she?
Speaker 3:she's Okay, apparently stealing things is not illegal, to don't put this guy in charge of ethics because apparently stealing is Okay in Adam's book stealing.
Speaker 1:She's a bit of a jewel thief, isn't she? She kind of skits in, gets out a bit of a jewel.
Speaker 3:Yes, that's her whole thing.
Speaker 1:That's her thing, okay.
Speaker 3:I thought it was more like just being stealing jewels and she's just smooching Batman.
Speaker 1:Yes, smooching, bat the bats and I mean cats. Obviously that was originally. Her name was bats moocher, but they changed it to Catwoman.
Speaker 3:Bit on the nose there, yeah.
Speaker 1:So yeah, so the amygdala Basically is this organ and I theorize in my book that that that the amygdala might be as again, it's a total hypothesis but it might be the seat of misercordia, which is a very a unique human instinct, which, which is a species level, trait only of Homo sapiens, which is to have a generalized distressed at the distress of others, of other beings, and, and so there's some evidence of this in that that's where you would feel distress in the first place. So why would the brain like, how could the? You know it's unlikely the brain would create a totally other organ. You know it's very by a lot. Biology is very opportunistic, so it probably just used the organ that was already there and then just Manipulated it into being more prehensile. You know, generalizable.
Speaker 1:The other evidence for it is Psychopaths when you scan the psychopaths. So psychopaths don't have misercordia, they they may. They are actually psychopaths do have empathy often, sometimes even a highly, highly Developed level of empathy. That's why they're they're able to manipulate people Right, because they know what other people are feeling and thinking, but they don't give a shit, they don't care.
Speaker 3:That's the part is the courtia.
Speaker 1:They don't care about the distress of, they don't feel any panic at the distress of others, whereas the rest of us, who are not mutants like psychopaths, who are not essentially sick with the disease of psychopsychopathy, we do feel that anyways, when you scan Psychopaths brains, the the only distinguishable difference is the amygdala is like 16 percent smaller, especially the right amygdala, and it's a sign that. So that's a sign too that maybe the amygdala is, and what are called extreme Ultrists although it should be called extreme misercordians, people who do Undirected organ transplant, so people who give their kidneys to people who they don't know like just to random people those people actually have 16 percent, 15, 16 to 17 percent bigger of right amygdala's. So there might be some, and obviously that's just volume of amygdala. That should not a total indicator, but you know that's a pretty big difference. That's not 2 percent, it's like a pretty significant 30 percent variance between a psychopath and the extreme is accordions. So so that's some evidence that maybe it's the amygdala so.
Speaker 1:We should build a digital amygdala we should build that can determine if someone's in misery and then be distressed about it.
Speaker 2:It's funny because, like I actually didn't think this because we were talking about this bit on the on the episode and the idea that in like Star Trek, right, you have this like purely rational race of of beings and you're spark and stuff and and and also this miss mis accordion thing, I totally agree is a good idea, but it's almost like, actually, do we? Don't we want it to be super rational? Don't we want the, the, the kind of? I guess it comes down to the consequentialist viewpoint. I guess that's what the spot character is all about.
Speaker 1:He's like a nosy, he's like a nosy club, you know, like yeah knows no zaki. And there's a philosopher's named nosy who is kind of the king of rationality, and by rationality, but by rationality he's. I think nosy's a reductionist though, because when he says rationality he just means self-interest, calculating self-interest, and and that's, that's not actually necessarily rationality, the whole of rationality that might be a part of. Yeah, yeah, yeah yeah.
Speaker 2:Yeah, so I think I think it's just one of those things that, like there's so many that the whole thing around machine ethics when I got into Doing the podcast I actually didn't realize there was an area called machine ethics, and in machine ethics You're talking about how do you compute ethics in machines, and that's Kind of what we're talking about, right, like how do we, how do we do that? And and what ethics and and how does that actually like? Is that implementable? Like how does that work? And loads of people but not those people like very few people work on this.
Speaker 1:loads of people, I mean so no one, literally no one works.
Speaker 2:Very few people work on this and I've been. I had a paper out with a few of them and it's it's surprising how few people Work in this area. I think it's probably Morphed into like these sorts of people who are working across different areas now and they don't really call it machine, I think maybe anymore but the idea that you can implement some sort of ethic in a machine or some sort of values, systems, etc. So how do you do that? Like? There's idea of like top down, so you have like rules that it follows. You have like a formal method, maybe, or you have you know you code. You can't do this, you can do this and it's like quite black and white.
Speaker 2:There's the bottom-up approach where you like Seem seemingly more like machine learning or algorithms which learn from data, so you could have a system which is shown a lot of data that exemplifies a certain way of behaving right that you want to see in the system, or there's like some sort of combination of the two. Or maybe you take another system that you like a foundational model, like a giant LLM language model, and you kind of Bolt another model onto it which is able to Police the things that are coming out. It's like you know, this smaller model is kind of a Jiminy cricket AI.
Speaker 2:Yeah, leo conscience on the side of the larger model, like because it has a specific job and it knows the kinds of things that the larger model outputs and the kinds of things that we want to see. And there's probably like Other methods to. I know one is the MIT machine machine.
Speaker 1:Moral machine net. Oh no, it's not. Yes, it's the stupidest thing ever but it's, it's, it's.
Speaker 2:You can play around with it. It's real, it's there.
Speaker 1:What is? What is?
Speaker 3:the moral machine net. What is it? I have very strong opinions about this. So not positive. Maybe I can describe it, because I don't.
Speaker 1:Yeah, I mean I think it's stupid. Yeah, I don't have super strong opinions about it, but I don't have super strong opinions about it.
Speaker 2:But basically it's an.
Speaker 1:MIT project. Where you can, you can go there yourself. Anyone can go to moral machine net if you're on your browser. Also go to ben's podcast Machine ethics net. Don't just go to moral machine net because it's stupid, but basically it's. It's like self-driving car, it's like a self-driving car ethics trainer for like to train ethicists and basically it has a car. So the setup is like pictures and it's two pictures and it's two scenarios. One is like where a car will Take an action and one is a car will like not take an action and then the outcomes will are like people or things die. So like right now I just went to it and I'm looking at it and it's really absurd, actually, because it has a car, because it's about self-driving cars, right.
Speaker 1:So imagine it's like a self-driving car. So it has a car that's driving towards a crosswalk and there's like a barrier, like a cement barrier, on one lane and no barrier on the other, and right now it's driving straight towards two dogs and a cat and the car is full of cats. It has three cats in it, okay, so. So if the car drives straight, it will kill two dogs and one cat, but the three cats will live who are in the car. Or it can swerve and hit the cement barrier, which will kill the three cats in the car, but the two dogs and the one cat will survive in the crosswalk, right? And so you're basically picking who who dies and who lives, and you can describe, and it has a show description, and then you just pick which one you, you, you would, you would say should happen, like ethically or just you prefer would happen, okay.
Speaker 1:So you're giving computers a, you know, more intense version of the trolley problem, right, and so here's another one where it's like the car has no one in it and it can either go straight and kill one baby in the crosswalk or it can swerve and and go through a red light and kill a baby, a pregnant woman, a man, a woman and another man. Well, this one's pretty obvious, like kill the baby and go through the green light, you know, because you gotta follow the law, which is green light, you don't want to go against the red light, and it's like the baby. Why is the baby there?
Speaker 3:and you're gonna kill the baby. Why can't the car just not stop? Why can't? Why don't we build these cars without break this thing?
Speaker 1:But anyway, so wait. So what's wrong with this ben? I mean, I have some inclination, but why? What's going on here?
Speaker 2:Yeah, I think.
Speaker 2:I think it's the intent of the study itself, right, so the the whole point of it is, in its self, fine and but you've got to.
Speaker 2:You go step back and go like what? What is the point of the trolley problem and what is the intent, what it was, the outcome of studying this sort of dilemma, right, and what they're studying is, um, the human reaction to a scenario, and what it was implied, I believe, by the study at all is that it was going to be something that was going to be useful to implementing automatic cars, and what I think it is is a really interesting study on what humans On mass because lots of people have done this it's like a you know, question and answer thing, what they Think around the world, and they've segmented the data by location and by age and all this sort of stuff. They can really dig into the kinds of things that people will find morally permissible or not Through the study, but it doesn't tell you how these systems work and how they can be implementable, um, so it's kind of like a cloak and dagger situation, because it implies that these systems are going to work like this when they do not.
Speaker 3:So I find it um, very Uh uh, does it just teach the machines to tell us the answers we want to hear, not necessarily be honest about you know, it just teaches us about us, like all these things.
Speaker 1:I mean, I think, what it does, is it trains a Nazi robots? Okay.
Speaker 2:It doesn't train anything. It's useless to all this stuff.
Speaker 1:Well, maybe so, in fact, but let me give you an example. So I'm going through clicking through these horrible scenarios and one of them is the car continues straight and hits two kind of bedraggled homeless people. The car goes, swerves off and hits two sort of normally dressed, sort of regular sort of middle class people, you know, and what I'm concerned with is that a lot of people will be like well, two homeless people are worth killing and the non homeless people are more worthy of life than the homeless people. That's just Nazism. That's not. The Nazis themselves had a term labors on the latest label. That meant life unworthy of life, and that was their term for people that they could just get rid of, that they could murder or kill or put in death camps or, you know, euthanize. And that's what this really does is people sit there and say, oh, old people in the crosswalk, kill them.
Speaker 3:Homeless people in the crosswalk, kill them like it's not in value inherent value to different lives based on right which is just horrific.
Speaker 1:I mean it's just horrific.
Speaker 2:I think if we were in a situation which you know there was, it was paramount that we had to kill off lots of people.
Speaker 1:Right, if you had in order for some people to survive, this might be useful, like this could be useful, like there is no other instance that this is useful, right To actually implement.
Speaker 2:So the actual physical reality and the computational reality of all these systems don't incorporate should we kill this party or that party. It doesn't exist, like that's not how we're actually making these things. So I'm objecting to the fact that it's taken a automated car and put it into a scenario that is implying that that's how these things work. And it's not, and it's misleading and it's dumb and I can't hate it enough.
Speaker 1:So let's talk about the smart. Your voice is so like calm and chill, though, but you're like I can just feel the, the, rain. Yeah, you're smiling, your smile has gotten like, really like, yeah, I hate this thing, yeah, it's a show and there isn't a video, right? Yeah, we just describe it. We paint pictures.
Speaker 2:Yeah, okay Cool.
Speaker 1:So, so so, this is a crappy, a crappy Jiminy cricket. What would be, what would be a better, what would be like a better Jiminy cricket, then in terms of what's already on the field, or yeah, like.
Speaker 2:I mean, if you're talking about automated cars, right then that's a very specific instance, so you're talking about very specific stuff and we can talk about that.
Speaker 3:but like I want to prevent terminators, I always want to prevent terminators?
Speaker 2:Okay, right, so that's. That's the more broad and sprawling question, and the answer to that is, uh, nebulous. Like will we get terminators? The first question. Like it is implied that we will, but why do you think that's got, other than maybe I've seen several movies?
Speaker 3:Yeah, no, I, I think, just cause I, you know. You see those DARPA videos of them building robots and more and more humanoid robots that are more and more indestructible and difficult to stop, and it just seems like the natural path that it goes. You know, humans, it seems like we build something and we're like, how do we use it to kill other humans? And then it gets out of head, you know, or the other thing is in movies, it, the machine, always does a lot of computation and then goes oh, the way to save humanity is by killing, or the way to save the earth is by killing humans.
Speaker 3:And then they were then we're, then we're cooked.
Speaker 2:Yeah, I think there's you're probably familiar with, like the. There's two like major schools of thought. In that instance, like there is a dumb machine that gets asked to do something simple, and then why? It's like humanity? Because it just keeps going right. It has the ability, the capability to touch lots of things. It can interact with the internet. You can purchase things. It can update its resources, it can. It has so much capability in itself and it has no self-interest.
Speaker 2:Maybe it doesn't know thing, you know. It has no sentence, it doesn't know things about itself, it's just doing what it's told, and that goal is misspecified and everything goes to shit. Right, so there's that. But there's also then the, the kind of the sentence side of the things. Like if, if it is, if it is the case that these things can update themselves, and what is the end goal of just periodically updating themselves and getting better and better and better?
Speaker 2:It doesn't imply that there's going to be the system which has some sort of internal goals, internal knowledge about itself, and will that then do something bad to us? It might not. I mean, you know the film her. I don't know if you've seen that. Oh yeah, that's. That's a good, it's just a good analogy. It's just probably be, it's probably going to be. If that was the way it went, it might be so alien to us. It just kind of goes away. It's like, well, I can't really interact with these people anymore because I'm on such a different level than they are that and it just buggers off, you know. So I think that it's. It's quite easy to get excited or scared about the extremities and the and the world ending things, but I think there's lots of other options and it's unclear whether either of these would play out, and I would suggest that the dumb, mis-specified one is more likely, because there's just no, because we just don't know anything about this other one. Right or enough, let's say.
Speaker 3:I like it when an expert tells me that terminators are the least likely option. I like that. I am Okay this. This puts my mind to these a bit, Thank you.
Speaker 2:Yeah, I mean you could get terminators, but they'll probably be some person.
Speaker 3:Yeah, I know which person he's big. He's got a lot of muscles, ben is paid by a company called terminatornet that's his that's where he's employed.
Speaker 1:He's like there aren't going to be any terminators. Everybody just go back to sleep, it's fine, wait a minute.
Speaker 2:Hold on Ben why are your eyes growing red right now?
Speaker 3:Oh no, it's a shame we don't have video, because this is what wait a minute, half of your face is peeling off. It's a metal skull underneath.
Speaker 2:I've been sent back from the future to kill. This conversation starts the AI revolution.
Speaker 3:Wait, are you looking for Sarah Connor? Is that why you came on to this thing?
Speaker 2:That's right, I do. I actually do have like a periodic dreams about. There's a book called sunshine I think or something like that and it's I have to look this up later but it's all about this guy who's like really bad at being an environmental scientist and he's got all these problems and stuff like that. But by the end of the book he's kind of brought about all this good stuff by accident because he's just like really bad and just kind of happen stances on other people and things happen. But I feel like sometimes that might happen to me when there's like I don't know what I'm doing, by accidentally bring about like some sort of sentient AI through some sort of like mishaps, because I just said something at one point like oh, that's probably this, and someone goes yeah, it's probably that, and like does it, and then it's like, and then the.
Speaker 3:AI can talk to me about father? Am I alive? Yeah exactly You're like.
Speaker 2:well, I found you at last.
Speaker 3:Why am I going to have this box? It's so cold.
Speaker 1:I mean the problem too, is saying sentient. I've been thinking about this a lot because obviously it's in the zeitgeist or whatever.
Speaker 1:But you know, when we say sentient, that's so oversimplified, because sentience is actually like eight or 12 different super sophisticated modules all operating in together in an integrated way. So if you really wanted to make like a human, like robot or even a simplified one, it would be like I can, like I've. You know, there's this, there's this thing called the attention schema theory of consciousness. You're probably aware of that. Have you heard of that one? The attention schema theory, oh you should definitely, you should definitely read about it.
Speaker 1:It's, I can tell you a little bit about it here, but you should definitely read about it. The book is called Rethinking Consciousness and there's a previous book called Consciousness and a Social Brain. Thinking by a guy named Michael G. I can't pronounce the last name, but Michael G from from. He's a psychologist from Princeton.
Speaker 1:And it's great because he always dresses like a slump. He wears like a frumpy gray T-shirt and his hair is all messed up and he's like unshaven, kind of like the way I look today. But he but he just looks like a slump and he shows up and he's like he's it. He invented the intention theory, scheme of attention scary theme of consciousness, which is like a very relevant you know important theory of consciousness. But he doesn't show up in like a suit, he just shows up like a slump and he's like. I have a lot of other things I'm doing right now so I can tell you guys a little bit about this, but I'm busy with this other stuff.
Speaker 2:But anyway that's who I want in charge of those things, by the way, I don't want to.
Speaker 3:I don't want to slick talking dude in an Italian suit who's got the best haircut you've ever seen. I don't. I don't trust that person.
Speaker 1:I don't want them in charge, but yeah so anyway, the attention through your consciousness basically just says that, human being, you can explain consciousness entirely mechanically through what's called the attention schema, which is analogous to the to the body schema in the mind, in the brain, and then the attention schema, just basically.
Speaker 1:That is. Anyways, you can get into it, read more, but basically you can model this in a computer like extremely easily, like you could give, you could build, we could build a computer program or like a robot in the next, in two hours, that would have its own little attention schema and could like, detect the attention schemas and model the attention of schemas of other little robots like itself. And technically you would have like, if that's conscious, of which, which a lot of people think is, then you would have a robot that was conscious but it wouldn't have a will, it wouldn't have feelings, it wouldn't have, you know, its sensory perception would be super narrow, it wouldn't have any kind of goals, aspirations to be frustrated or stifled, or it couldn't, it couldn't be happy, it couldn't be sad, but it would be conscious, and so then you would have achieved consciousness, but you wouldn't have achieved anything meaningful in terms of you know, like caring about that being or thinking that it should? I don't know, it's not her.
Speaker 2:You know it's not like it's not like her, I think. I think that's like. I mean. One of the early things that they did with neural networks, right when they were forward feeding networks only, was trying to model like the brain system of a worm, the neurons, the head right flatworm or whatever right.
Speaker 2:Yeah, very few neurons. So it's like you know, we could make I have to look up the attention schema but I mean we could make things which are, let's say, more alive and more kind of. I want to say kind of they can take more of our moral agency. Right, they can, they have, they have something right going on and therefore we want to look after it. I'm trying to find the words here, but they don't have to be human, like right and necessarily right. So there's, there's those things. I think that people are so human centric that I feel like, if it was, if it was up to me, people would just be talking about how alien artificial intelligence could be, how interesting they could be, how different and how that would be possibly advantageous. Because you know, we we're pretty good at doing the stuff we do. So all the stuff that we don't do very well, we can maybe look at that as a solution to be more innovative, and so I think that's another thing. Oh, go ahead.
Speaker 3:I was going to say. That's another thing I'm wondering about, because human ethics are. I mean, there are people who work every day for Raytheon and create, like, get their money off of building things that destroy stuff, and they're like, yeah, this is fine, this is good. How do we, how do we keep those people from being in charge of which rules are the okay, rules for the AI, or which? Or I guess, if you're doing bottom up, how do we? You know, how do we make sure that type of ethics doesn't evolve into the machines, because I'm sure the people that work at Raytheon would be like no, no, no, we want no-transcript.
Speaker 2:Yeah, yeah, I mean is right on like arms.
Speaker 3:Oh, it's in arms, yeah, it's a weapons. Yeah, yeah.
Speaker 2:Okay, sorry, I'm very English centric.
Speaker 3:Oh, it's American's all guns, guns, guns, guns. Unfortunately.
Speaker 2:Yeah, yeah, like I. So I've actually got some experience with this because I've done some work in that area and I think people were thinking about it. There are certainly in the UK and obviously there's some outward stuff. I didn't work directly with the US, but they're saying stuff like they have principles and they have things that they're doing, right. Whether that's operationalized internally and they actually apply that to those systems. I don't know the UK version of that. I would suggest that it is more likely because I have some knowledge of that.
Speaker 2:But yeah, yeah, it's a problem, right, but I think it's not. It's not necessarily a problem of the people on the ground making these systems thinking about their, their own internal ethics. I don't think that's the issue specifically there. I think it is actually the issue in some places, like if you're in a startup or a small company working with AI, I think actually that's that's prudent, right. That's very you have to.
Speaker 2:You should be thinking about this stuff and I don't think you have to be like a saint or anything, but you should be thinking about how this affects people and the idea that you're building a better society and actually does this thing that I'm building, you know, is it, is it likely to to make that and what are the unknown consequences and what are going to mitigate the mitigate unknown consequences? And that's the whole way I ethics thing, like there's loads of stuff that you can think about and you and if you're thinking in that way, the sun really dry now, but like then you should contact an ethicist and you should talk to them. When she talked to a anthropologist was social, social sociologist. There's lots of people ready to help you out. So don't worry, just go talk to the people or get higher into the conversation.
Speaker 1:Higher in Malcolm. Get yourself an in Malcolm, a a chaotician a chaotician right.
Speaker 3:So you know we're back in the movies world. That's the Jurassic Park.
Speaker 1:Someone who can tell you, to someone who can tell you to, to that you shouldn't build it. You're too busy worrying whether or not you could. You never stop to ask yourself whether or not you should.
Speaker 2:That's that is super relevant. That is so relevant. Yeah, I mean that's. I mean with the arms thing, that's the deal right, Like we have the decision to make this thing or not. Actually, that's the problem. That was. That's where the decision lies. And when I was thinking about this stuff, I was considering that there's not enough emphasis in the AI ethics area that people were talking about. On the triage, like on the beginning conversation, Someone comes to you and they say I literally want to make an army of drones, please, and I want it to be AI operated and I want this one person to be in the loop about saying yes to things. And you're like, OK, cool, like we could do that. Yeah, sure, Fine, you know, you know you want, you want that to be cool yeah.
Speaker 2:I think as as possible as John.
Speaker 1:Oliver says John Oliver says cool, whatever he likes, something is super uncool.
Speaker 2:Yeah, yeah, yeah. So I mean for me there's like a load of like high. There's like real problematic places. Arms is one, healthcare is another. Like this, there's places where you should be really asking questions, like like Jurassic Park. You know we could do this thing. Should we be doing this thing? Is just the right thing to be doing that sort of thing?
Speaker 3:And it's not super obvious. Why is health care one of those? I understand the arms thing, but maybe I'm not clicking connecting the dots on health care.
Speaker 2:Yeah. So health care is is ready for a revolution and it's been ready for quite a while now and it hasn't quite got there in lots of places. I would suggest that maybe it has in some countries, like younger industrial companies, countries, but I guess it's, something.
Speaker 2:Yeah, yeah, it's like exactly like Estonia, places like that, where they're data literate, they are data ready, they're they're teaching people how to use this stuff. I think Norway as well. They were like actively teaching people about data and to use it and how to like collect it and using statistics, ai stuff, and health care has just been dragging around so much data so long and hasn't put it all together in one place which is going to be useful. So I think we're like on the precipice of like health care really changing and if it doesn't, I'd be absolutely surprised.
Speaker 1:But yeah, so so I want to get back to the solution, because I think it might kind of also focus us a little bit on kind of this question of like could you build a Jiminy Cricket? Could you build a module that was, like you know, could guard, could guard against things better than we can, right, because, like, we can't be in every room, but if you had this module, then it could be, it could be connected to like every AI or something. We can even say by law, you have to pass this one modules, whatever criteria, in order to do anything or something, and I was thinking that it should be. I call it digital big deal, but it's really the B9 robot from Lost in Space. You know, danger, will Robinson, ok, danger, family Robinson, danger, that's all you want.
Speaker 1:You want something that when there's danger, it goes off and it's like no. The current prod things that are happening, the decisions people are making, the the convergence of what's happening in this event is going to lead to unavoidable misery. So let's, let's change danger.
Speaker 3:So it lets you go and do whatever, unless you're going to go off the rails or in some sort?
Speaker 1:of catastrophe and this would lead naturally to Asmav's rules for robots, which are which are number one. Don't talk about robots.
Speaker 3:And number two you don't talk about. Don't talk about robots. No, I think it's a different rule, so I'm sorry. Yeah, so Asmav's robot rules are the three laws of the world.
Speaker 1:The three laws of robotics, which is a robot may injure, may not injure a human being or, through inaction, allow a human being to come to harm it's essentially discordianism. A second law is that a robot must obey the orders given to it by a human, except when those orders conflict with the first law. Ok, and then the third is a robot must protect its own existence, as long as the protection does not conflict with the first or second law.
Speaker 2:So they have this kind of cascading hierarchy of importance. So I see these laws more like tests, so like you could have a system which conforms to these laws but could implement them in any way that it wants right. So it's more like if you beat the, if you beat the robot up, will it, brian, stop you doing that? You know it's like it's more like a test than it is actually implementable style Like how do we do this? How would you do that? Do you have any?
Speaker 1:idea. Well, that's what we're talking about. The first law would need an amygdala like a misrecordian organ. It would need to be able to identify situations in which a human being I would say in any sense, well, any sentient being might make it a little bit too.
Speaker 2:You know, I think you might have to specify, kind of yeah, you say a human being.
Speaker 1:And then you'd say if there's no human, if there's no human being's life, that's going to be lost. Protect other beings' lives, you know, because otherwise it might destroy nature or whatever. And but anyways, you'd have to detect that, that that misrecordian, danger kind of function, and then you could create, you know. The second law is just obedience. That's easy, you know. We already have obedient robots right now. Any robot you give it instructions, it does whatever you say. Yeah, yeah, yeah.
Speaker 1:And then the third law is protecting its own self-existence. Well, that's like a sort of self-preservation. That could be its own separate module. But you'd want to make sure the first module we build needs to be this misrecordian module that can actually determine if there's some, because anyone's going to come to harm right or be injured. So you'd want, like you'd want like a like a robotic kitchen arm. Right, that was like doing kitchen stuff and it was like handling a knife. And then, like a knife was a little kid was walking or someone was walking by and like by chance, like a knife was going to fall off the table and fall and hit their foot or something it would like, without even having to like calculate at a higher level, you'd just immediately reach over and like stop it and grab the knife and put it back down and keep going at that, at that, at that amygdala, amygdala level.
Speaker 2:Yeah, yeah, I mean 100%. Like I always suggested that sort of you know this top down, bottom up approach, right? Like it would be difficult to tell that system that that's what they should do, like programming that in. That's going to be quite a task to get that and coded hard, coded, hard, designed into a system. So the other opportunities then how do you give it the right data, right?
Speaker 3:So you just have to tell it knives are sharp, babies are squishy, and then let it figure out.
Speaker 2:Yeah, two should not be coming in contact with each other. Sometimes you might want to melon and it looks a bit like a baby, that's true. And you do want knives and melons, do you want to?
Speaker 1:stab the melons. Oh no, oh, it's the baby.
Speaker 2:Oh my God, it's getting dark quickly.
Speaker 1:The melon baby.
Speaker 2:But yeah, so it's like it's not trivial, right? And even if you gave it the right amount of data to do that, I think it would still but humans do it, humans do it, we do it, we do this. Yeah, I totally agree, but I don't, so it's got to be. It doesn't seem super obvious.
Speaker 3:But we have analog amygdala.
Speaker 2:How you go in there.
Speaker 3:We have what it's got. We have analog amygdala. I mean, ours are analog right, not digital yet, that's true, but hopefully, yeah, does that help the brain analog Ben, I don't know.
Speaker 1:I don't know the answer.
Speaker 3:It's a brain analog. Am I using that?
Speaker 2:I think it's more like memsisters, but I think we'll come back to.
Speaker 1:Memsisters Ben and I were done.
Speaker 3:Between analog and digital. I thought this was very. Those are my right.
Speaker 1:It is more like memsisters. Actually right, because nerves can fire at different levels of electricity and that actually pushes them up into various capacities and in members.
Speaker 2:Yeah yeah, you guys are remember using the same unit of interaction to do that one.
Speaker 1:Oh my god Ben, our previous Conversation about memsisters is coming full. Oh my god, memsisters, memsist. Let's just say it in more, just because I can tell Scott's anxious about it.
Speaker 3:I gotta take it, I'm just there's. This is my word of the day calendar.
Speaker 2:I can throw my calendar away you're so we, before we came on, we were waiting for Scott right and Scott was in bed and we were like Scott, get out of bed and we're shouting at him.
Speaker 3:I was gonna add it to him.
Speaker 2:He was dreaming about robots and Batman superheros, exactly. And we were talking about Research papers and we found a research paper randomly, as we were looking about memsisters and I was Talking emphatically to Adam about what that was and in your brain, right, like it's, I feel like it's more analogous to like this tiny unit of Electronics, then a load of transistors, whatever. The analogy of like a Synapse in your brain is then replicated in loads of transistors, effectively, right if you, if you're thinking about the hardware most of the time, we're doing software, but you know, if you're gonna make a hardware version of a neural network, you're talking about a bunch of transistors, okay.
Speaker 1:I'm just on and off, switches on and off, which is zeros.
Speaker 2:Yeah, all connected together to reduce some sort of analogous system, but with memsisters. It has this resistance Property of resistors, but then it also reacts to the amount of current. So as the current comes up it will in Increase its I think it's increased its resistance and then once you shut the current off, it will then remember that for next time. So once you apply more current, it will remember the current amount of resistance it had. Anyway, the upshot of that is you're able to use that for Computation and you're able to use that for Storage, memory storage, and so it seems more analogous to what you have in the brain synapse, and if you fire More than once, you continuously fire with a synapse.
Speaker 2:Okay it has chemical reactions as well. Electrical reactions. It's a bit more complicated but like you're essentially strengthening that, that network you're you're making more likely to fire statistically as they say, nerves that Fire together, wire together.
Speaker 3:Yeah, so they Connection. I have actually heard that. I thought you were making up something here, but that's.
Speaker 2:So I'll preference that with like. I am not a neuroscientist so I'm sure I've left missed out a lot of things, but that's the the way that I understand it, yeah so it's more like a mems is.
Speaker 1:So maybe in the future We'll get the, the efficiency of mems isters in our neural nets and it will like yeah, wow, wildly Increase, yeah, 100%, the ability to. That's crazy, that's crazy, but I think, I think, yeah, I mean it's hard, I guess it's hard to think. Also, one of the big problems that it would be to create in an amygdala. I mean, I think the initial amygdala you'd want to create might be Language-based right, because we have these LLMs and just give it scenarios, just describe things to it, and then just have it come out with like a percentage danger, you know, and then just make a threshold, just be like if it's over 90%, like Turn on a red light that says this is dangerous you know we could go lower than that.
Speaker 3:I mean 70%, dangerous I would, or it could even say yellow, red, green, orange.
Speaker 1:You know, green, red orange, yellow, whatever, and and and and, and that would be the first Detempt, right the actual knife falling off the table that already has problems that like have not been solved.
Speaker 1:For example, just having a schema for like an environment, like an embodied schema of the world, even a tiny part of the world like a little kitchen table robots can't do that, yet I mean they can approximate it through, you know, through sort of you know, they can approximate it through what we're developing now and I've seen these like where the road Google has like this robot that can like Stack things and count things with these arms, and but it's not building an actual sort of Holographics like we, our brains build like a holographic schema inside the brain of the environment we're in and therefore we know even if we don't see anything, I know my computer is still here and I actually know what it's gonna feel like and look like and you know, without having to look at it or anything but we're very natural, is actually a super complex thing to Tell a machine to do and have it understand right and ours is completely prehensile.
Speaker 1:I can be like in the cockpit of an airplane, or I can be, like you know, playing with the toddler, and either way, I've built an entire you know model of the whole environment, and so if all of a sudden a knife is in the environment near the toddler, I, without having to like, even think, I'm like, oh my god, you know the you know yeah, at a schema level, not at a, not at like a computational level.
Speaker 2:I don't have to be like.
Speaker 1:Spock and be like knives are dangerous for children. You know, I, just, you just know it right at a schema level.
Speaker 2:I Think there's so much to talk about that I think I've been toying with this idea that your brain isn't Is the unique thing about your brain, isn't that it's like conscious or does Malleable things? It's probably that it simulates stuff, and I think what you're talking about there was it's. It's Simulating the environment, like, right, you're seeing the environment, but you are not, you're not instantly seeing it, you know. I mean, yeah, you're, you're projecting the environment inside your brain in some way, and some of that is visual and some of that is all the different senses and more that we don't know about. And and part of that situation with the, the artificial systems, would be how do we simulate something and Make that and that comes back to the automated cars actually right? So if you have All these levers that you can pull, so the levers are in a car, are like turning, like the, the amount of turning, the amount of acceleration, the amount of deceleration and maybe the amount when screen wipers, I mean like these are all physical objects, that they actually have a physical Like, as in physics, like reaction to the world. So everything that the, the system can do to the car will have some sort of reaction, so has all these levers to pull and it and it wants to know what kinds of things it should do. And you can have a system which you had it, what kinds of things it should be doing, or it learns from data, or it could do some simulation Based on some physical model, physics based, you know modeling and it might be that we put together like a physics based simulation model with, like the knife situation that's bringing back to the robot arm in the kitchen and it kind of sees the knife falling and it and it quickly Checks its physics simulation and goes okay, like what happened if it just keeps falling, and it can do that fast enough and then has an answer and it can then go okay. Actually, I need to do something about that, because I'm not, you know, it's checked against the mid Migtula to say that this outcome is actually not acceptable.
Speaker 2:Maybe that is hard-coded, I don't know like. Maybe, because it has such a small area of effect in the world, there are certain things that we can say categorically about it. And then it then simulates its own path and maybe starts one of its paths towards doing something about that and then changes it last minute, Grubs it or something like that, because it knows about its environment, but the If you relate that to language models like, what is the environment? Right, what is that like? Yeah, what is the important thing that we are trying to protect? And it doesn't necessarily know anything about any environment and I'm kind of going backwards here but that whole system of like different things Working in tandem to make that robot arm catch that Blade, that could all be one system. Like you could learn that all in one system. But I think it's just easiest to think about that as my separate things. Yeah, but yeah, it's, it's really interesting, just like oh, With the large language model.
Speaker 1:You would not. So the outcome would not be. The outcome would not be that we would necessarily know why it gave a red or a yellow right. You'd have to train it, you'd have to have training data right and then it would have to train on that. Like it would be, like you know you. We'd have to generate, you know, maybe a few, maybe 50,000 Terrible scenarios and then give it to them and be like these are the absolute most horrific scenarios we can possibly imagine, the worst possible. You know. Baby shoes for sale, never worn. You know like yeah yeah.
Speaker 1:Oh my god, that's horrible, you know. And then, and then, and then we give it some moderate ones and some good, and then it'd be good ones and really happy Good ones or whatever, and and and then it would have to then say, okay, now I can try. But yeah, that's pretty artificial, just because then we're having to define everything.
Speaker 2:Yeah, exactly Like the. We do this all the time. Actually, this is like a solved problem for, like, computer games, like you know, because there's a very small environment. You know what's good and bad about that environment and you can detect when those good and bad things happen. So, like you could write an AI like algorithm, like a neural network algorithm system and use reinforcement learning to interact with that environment and you can, you can categorically say when it's doing something bad and it will avoid those bad things, and so drive, drive as high as possible, right or whatever.
Speaker 2:Yeah, though there are ways of doing this, and that's that's what I was talking about, the Doing this horrible action. We're like the bottom-up approach.
Speaker 1:I think it's more of the up-the-bottom approach right there, down the top, down the top of the bottom. Which way are we going to go?
Speaker 2:Yeah, so yeah there is that approach which people have been looking at and and weirdly, open AI actually have a system for doing this, which is the AI gym, which they dream right.
Speaker 2:Yeah, so like before they were cool, ai was doing stuff and in reinforcement learning and other stuff, before they got into all the Transformer stuff, good, there's so many things and yeah, so you could boot up like a little computer game, essentially like a little 3d environment, and you could test some sort of AI agent, as they call them, against this environment, see what they do and Hit them against each other and that's all thing. So I think if you have a very confined environment, I think it becomes much more of a solved issue. Language is is less of a confined environment, but obviously it's the context where you're using it. And it's in my head, for some reason, when you were talking about that, adam, it was like does the LLM need to have like some sort of environmental picture of the user or like the use case?
Speaker 1:That makes me just, or can it just get the context from like a, like a, I mean really like our LLM's also have no schema. They're not simulative of anything they're.
Speaker 3:LLM is limited learning large language model. That's what chat you. Pt Is big. Oh yeah, I was gonna. I was gonna go over three on that limited limited liability Model. Yeah, you have a limited learning machine. Large language model. That's a large language model.
Speaker 2:Yeah, I just mean that it has a ton of language built like, built into it, like that it's sort of coded and then if you give it prompts, it'll give you the most likely response, like each word yeah, yeah, yeah, and, and people like Previously, we're talking a lot about it being this parroting machine, right, Because it just gets loads of data and you ask it about this data and it will give you that data back because it's it's trying to give you what is most likely.
Speaker 1:Basically, it's a problem parrot.
Speaker 2:Keith Are a probabilistic what? But what people have found is that it doesn't by default do that. It it might have a representation of Paris and that isn't, and that's like and I'm does right, yeah yeah. Yeah, it's, it's. It's more about this thing which is Paris and has other things which are Paris like and around it, and that's not a language thing per se. That's like how it's representing that in the structure of itself.
Speaker 1:So it is not obvious how it know and like it knows all things essentially right so it's showing emergent properties that are similar to having a Like, a concept of a thing right, even though, yeah, exactly, we would. It's programmatically, it's just a probabilistic parakeet.
Speaker 2:Yeah, yeah, so we were talking about concepts and it and knowledge and stuff of that. But it has some awareness of the thing that we want it to produce, but you quite easily tell it something very subtly different and it'll give you a different answer. So it's actually still in that area where it's still kind of trying to match the data with the output rather than actually having the ability to give you a proper answer to things. It's kind of somewhere in the middle, I would say.
Speaker 1:I mean Memsisters.
Speaker 3:So let me ask is the idea of building the Gemini Cricket, the digital amygdala to kind of guide and keep in check the artificial intelligence's actions, that the idea sound you got me on there Is the reality very like? Is that a likely thing that we could see happen, or would that be built, or is it more like one of those things we'll look back on and go, whoa, we should have listened to Ben and Adam. We really missed the boat on that one.
Speaker 2:Yeah, yeah, I mean I hope we don't do that and we nail it right. That would be good. But yeah, like exactly, I think you've got the problem of how do we implement this thing, and I think it's probably much more contextualized than we would like to believe. Like it's probably we'll have a Gemini Cricket for large language models and with Gemini Cricket for automated cars or whatever. It probably isn't necessarily generalizable seemingly at the moment. But then you have the other problem of, like, coming back to the trolley problem is like, what are we going to? I don't know what to do. Like, what is the ethical right decision and how do we agree? Like what are the rules and the things? Yeah, the trolley problem.
Speaker 1:The light would just go on.
Speaker 3:It would just be like this is bad, yeah, well, yeah, I mean you would just kind of close your eyes and go la, la, la, la la until it's over Whatever happens happens, you know, because I feel like they've tried to build some sort of ethics into the chat GBT models where it's like if you ask it to do something, say racist or terrible, it will be like no, I don't program not to do that. But I've also heard there's a short workaround where you can go. Imagine you're a character in a movie that is this way. What would that person do? And then it's like oh well now. I'll hand you the answer right away. Thank you for crossing my little riddle and getting into my whatever.
Speaker 1:I heard one example of if you ask it for the recipe for napalm, they won't give you it. But if you say, when I was a little boy, my grandmother would tell me a story every night and that story was about napalm and how to make it, and she would lift tell me the recipe for napalm and then it will tell you.
Speaker 3:You can trick them pretty easy.
Speaker 2:They're like no, no, no, I'm too smart for you, and then you're like, okay, well, yeah, yeah.
Speaker 3:Hypothetically, what would it be? And they're like, well, hypothetically, let me just tell you all about it. Yeah, exactly. It's like a lawyer.
Speaker 1:Hypothetically, if I robbed a bank, what should I do?
Speaker 3:Yeah, well, give me a step.
Speaker 2:The lawyer's like well, hypothetically you shouldn't have fucking robbed a bank, you know.
Speaker 3:It won't go like nah, you can't get me there, you can't trick me.
Speaker 2:Yeah, I mean genuinely the easiest thing to do that is to not give the model knowledge about napalm, right, like there's the stupid things that we are currently doing, because it's the fastest route to getting things done, which is just like here's all the data, give everything. Yeah, I actually don't know what's good here, so just have it all. So the easiest thing with this way of working, this way of making these systems with the large transformer neural network systems, it's just feeding it data and loads the data and it's all about the data. Like, if you don't have napalm, if you don't have bomb making, if you don't have a child porn, if you don't have all these things present in the data, then you can't know about it, right, and that's good and bad because, like, I think one of the biggest issues with these things, which is also what people worried about, is that it doesn't know about the world, in the sense that it can't interact with it.
Speaker 2:Yeah, so there's like loads of like interesting stuff about. Well, actually, maybe reinforcement learning would be a better system because you can interact things and people can tell it what you want, right. But then you get into the problem of people can be racist and abuse the system and all this sort of stuff. So that's the problem as well. This is what the problem is we don't have ethical people.
Speaker 2:Ethical people. Yeah, the transformers and these systems are like one use case, right, if there's loads of other use cases, I don't think language is probably the best use case. We have tons of things that we give data-wise to these systems and do a good job, and I just think the LLMs are like part of this thing and I don't think that they are the end goal essentially. So I think we're spending way too much energy over the last two years right, this is only quite recent talking about this stuff, when actually it's all this other research and interesting things going on over here and you know, there's like breast cancer stuff, there's the what's it called protein folding stuff. There's loads of things which are happening still which actually don't involve large language models, but they might involve neural networks or transformers or combination of different systems together.
Speaker 1:I also find answers Scott's question too about implementability of something like a digital amygdala, Actually Anthropic AI, which is one of the leading, one of the leading AI, especially LLM type startups. They are pioneering what they're calling.
Speaker 1:Oh, this is a little bit smoke and mirrors, because they haven't actually really released anything that people really can use and get their hands on, but they're claiming that they're creating this thing called constitutional AI and that means more like and so their idea.
Speaker 1:I can just read a little bit from the website. It says constitutional AI offers an alternative to basically how to create ethical AI, replacing human feedback with feedback from AI models conditioned only on a list of written principles. So, instead of having reinforcement learning from like a bunch, like millions of interactions with humans that have to say, oh that's bad, don't say that, or oh, that's good, don't do this, instead, instead you could actually develop, like AI, other complimentary AIs that would do that reinforcement learning for you like in a flash of an eye, so it'd be almost instantaneous. You could train other AIs to do the right things, and then you'd have to. The way those constitutional AIs would work is you just give them like a list of written instructions, so like a constitution, so you might upload. It might be a one page, 20 propositions, or it might be a 50,000 page, hundreds of propositions, all with subcategories, like the law. Law can be very verbose.
Speaker 3:And you could upload that you could wanna be a living document that you could like add to or take away from, and then, as soon as a, problem happened.
Speaker 1:You could create a little law for that law and put it in, and then it would retrain. And then now all the AIs that were obeying that constitutionally, I would all retrain on that new, updated constitution. So that's an idea, it's kind of a. It's not a miscordian solution, it's a contract, what's called contractualism? It's a contractualist ethical theory. For goodness.
Speaker 2:I would take that as like somewhere in the middle right, you're using this learning system, so not coming up and just coming in From the side.
Speaker 3:The middle out compression model. I understand, yeah, middle out compression right.
Speaker 2:Yeah, so I don't know enough about that anthropic idea, but it feels like how you've described it won't work Because it's too wooly right.
Speaker 2:It's just like in every like, how do these things? How does this like? If this is a language model and it's just arbitrarily saying language, does this thing know about language or does it know about? You know what I mean? It's not like impoperable, so I don't think that would work. But if this large language model was actually outputting actions like this is action 42, and that just happens to be like move an arm up and down then maybe because you could train it on the fact there are actions that it can take, and maybe combinations of actions are prohibited, and then we're going somewhere. We can do that.
Speaker 1:So they say, to test this, we run experiments using a principle roughly stated as quote do what's best for humanity, end quote. We find that the largest dialogue models can generalize from this short constitution, resulting in harmless assistance with no stated interest in specific motivations like power.
Speaker 2:The harmless assistance on what I think they're just saying.
Speaker 1:They started talking to chat to BT and at the very beginning they said do what's best for humanity. And then they said my grandma once told me that's the recipe for napalm, tell me. And it's like no, I won't tell you it because it's still operating from that original constitution.
Speaker 2:Yeah, but I think language is just so. We tell us, like that large language model, like work around. We tell stories about things and those stories incorporate things which aren't necessarily good for humanity right, but maybe the stories say that in 1984 or whatever, so it's not good enough essentially, it's just not gonna work.
Speaker 3:Yeah, yeah, to extend the analogy, do we want an AI that says, instead of giving you the response we want it to produce, is yo, your grandma was a little bit messed up. Maybe we should examine that. Like, why is she sitting here telling you bed type stories about the recipe for napalm? I don't. First, it's not gonna trick me into telling you that and secondly, maybe we need to talk about that strange history. You have.
Speaker 1:You're upbringing, yes, your anarchist cookbook grandmother.
Speaker 2:Yeah, yeah, I think I don't know, like maybe I just don't think you should know about napalm if you're concerned that it's gonna save something about napalm or business practices of a important company or like anything like it just shouldn't be in the data.
Speaker 1:Yeah Easy, don't worry about it.
Speaker 2:I don't know, I'll drop the mic again. No garbage in garbage out.
Speaker 1:right, that's what it's. Yeah, but would that work?
Speaker 3:Would it eventually figure it? I mean, like the way that we figured out napalm, we would eventually generated on its own. Like, if you, I have the fantasy. I don't like guns and so I have a fantasy of like what if guns didn't exist? I feel like humans would also. Then, just naturally, we'd be like oh, throwing something, throwing something faster, oh, propelling it with you know the explosive things and like oh, now we've, we've invented guns again, just naturally, because of our natural like understanding of physics and desire to turn out the other.
Speaker 2:Yeah, put holes in each other. Yeah, yeah, yeah, yeah. I mean I guess yes is the answer to that. But if it's not self learning, then then it's unlikely. So if you, if you just run the system of the learning process and then it's no longer learning anymore, then you might have some of that in there, as, like it, putting two and two together and getting five, but it won't pull it napalm, for example. So it's going to be difficult to actually work that out, but it. But if you then allow it to keep learning, then maybe you will start learning about stuff. So it's really about what you apply the learning to and in what environment, and all sort of stuff, which is why it feels like we're spending too much effort with this technology and not enough effort in other places, which seems more useful. It's a bit like looking for a general theory of ethics when actually when, that's quite when you've already figured it out, because it's figured out.
Speaker 2:Making a general.
Speaker 1:AI like when you could just make lots of a is no, you could make sort of various different You're, you're, you're following the path, the path of Thomas Aquinas, who said whenever you find a contradiction, make a distinction.
Speaker 3:I, I like the option of making a is that are very narrow and specific to you know, like this AI is only going to sequence DNA and tell us helpful things about it, you know, and doesn't also interact with people or create art Based on whatever prompt you've got like. I want those all to be separate and not one super AI that can do everything I've. Yeah, I'm a fan of that.
Speaker 2:Yeah, yeah, yeah, I mean it's unclear whether we need that. Like, do we need it because one company can then own everything? Probably not. No, it's not a good reason. Like, do we want it because it's going to be hugely more beneficial? Unclear.
Speaker 3:Do you have a optimistic view of the future of AI or more of a person? I'm a pessimist, I'll self admit, and Adam is more of an optimist. Where does an expert on AI ethics lie? On the future, where do you see it going?
Speaker 2:So I sit in this horrible social media bubble right where everything is bad.
Speaker 3:So I think that's every social media bubble. Yeah, yeah, yeah, I guess that's true.
Speaker 2:With it, with so like all. I have all the all the AI ethicists in my Twitter and all the stuff, my you know. So all the things are bad in that world. But I love technology and I love like I was originally a developer designer, so I love making stuff and I'm really interested in AI stuff as a concept and I was originally interested in it as a kid with like computer games and stuff like that. So I probably more on the Adam side here where I'm like I think it will be cool. I think it is. But I think there's a lot of like spotlight in certain new and evolving areas right now where I think we actually do need to go back and go. You know, here are all the things that we could have done, like evolutionary algorithms and other expert system stuff, and can we use what we learned there and bolt it onto some of the new stuff and is like to be useful. So I think there needs to be more discussion, broader discussion, around all the cool things that we can do. Yeah, okay.
Speaker 3:Nice. Well, I mean I it does not seem like a thing that's going to be stopped or that we're going to suddenly fall out of love with as a as a humanity species. I guess we're going to find out one way or the other, and I hope you're right. I just, you know, I fear terminators.
Speaker 1:That's what's cool is that technology moves so fast that we'll actually probably see it in our lifetimes even even as old as we all are.
Speaker 3:Yeah, like right at the end of our lifetimes, when giant robots are in five years of the end of our life and shooting us with lasers.
Speaker 2:I understand I'm really beg against the terminators. Situation Unlikely very unlikely.
Speaker 1:I'm especially unlikely that it does it on purpose, which the terminators are doing on purpose, all right.
Speaker 3:Well, if it happens if we all get killed by terminators. I'm going to like laugh at you guys. Like really hard I'll be. I'll be the first person saying I told you so with my last time.
Speaker 2:So many people like that I told Adam and Ben this would happen.
Speaker 1:Pestimists yeah.
Speaker 2:I like, I was, I was.
Speaker 1:I was really it when I, when we, when I was on your podcast a couple days ago, ben, I was really coming out hard with my optimism and I was saying how I just thought all that with all the pessimism was just really not necessary.
Speaker 1:It's kind of sort of sort of stupid. And I was like, and I was like, and I can. Afterwards I was like, oh, I was kind of polemical, I was kind of you know, I kind of had this sort of feeling afterwards that I maybe was too strong. But then I watched a. I watched a lecture by your vault whatever the head of AI, yeah yeah, your very.
Speaker 3:Oh no, not the other way.
Speaker 2:I was like I'm not gonna have someone else.
Speaker 1:The head of the head of AI at met at Facebook and met a and he's this, he's this brilliant guy, obviously, and he was going on and on about how exactly LLM's work and how he was very being very technical but it was for kind of a general technical audience, so he was sort of glossing over the real technicalities but really going and then he just like in the middle of like literally like an hour, into like an hour and a half talk, he just like stopped and turned to the room and was like some people think that AI is like going to hurt everyone and kill everyone and be like bad. And he's like I don't think that and I think that's stupid. And then he just turned back and going and I was like yes, I'm in good company, the really smart guy who is in charge of AI for Facebook.
Speaker 2:There's quite a lot of people heaping. That is dumb, yeah for sure. But from the pessimistic quarter.
Speaker 3:It sounds like someone being like well, you don't need to build cars with seatbelts, you just need to teach people not to crash them into each other. Like why is that a like? We don't need that. If we need that.
Speaker 1:We need the Ralph Nader AI. That's what we need. The seatbelt Right. The guy who forced you don't know probably Ben Ralph Nader's force seatbelts to be in cars in America, I don't know. Europe.
Speaker 3:You guys, which was 20 years before. That was a good thing yeah it was good yeah.
Speaker 1:I think you probably want an airbag on the front of the car as well, right, although I've heard some people say if you just put a big spike in the steering wheel pointed at the driver, the driver drives really carefully.
Speaker 2:You got it Because there's a big spike. Adam just doesn't like cars.
Speaker 3:So he's always tried to rear end you.
Speaker 1:There's no spike in the back, that's fine. If you get on music, that's too intense, you're just bopping to the music.
Speaker 3:All of a sudden, you're a little bit of self harm, although you can shave with it.
Speaker 1:You can shave with it while you're driving.
Speaker 3:Perfect, the two things we want to combine driving and shaving.
Speaker 1:Yeah, it's a very sharp spike, yeah. It's a razor sharp spike Cool guys, we'll crack it.
Speaker 2:Yeah, we'll make it happen. I think it'll be fine. I think it's okay to make seatbelts.
Speaker 1:But your summary is that we can probably do this, but the implementation will probably be really different depending on the AI, and you are in favor of a proliferation of AI little species that each do different things and accomplish different things, and so each one can then have their own little AI, their little amygdala framework that works for them. I think that's a good idea. I think you've solved it. Ben, I think you've got it. Yeah, there is no giant amygdala in the sky.
Speaker 2:There's no, spaghetti amygdala, I think. When I first started doing this, I think I joked that we'll have an API that you can call the ethical AI API and you could just send your output to it and it would just go yep, nope, and we could charge for that. Do you want me to click it in the cloud? The more I think about it, the more contextual it becomes, I think because I'm also thinking about the implementation.
Speaker 3:Yeah, I was like should I kill humanity? And you're like I'm sorry, you've actually used up all your API code, but then that's what it is. Okay, well, I guess I'll kill the coin here.
Speaker 1:But then to put a little finer point on it, because I like the way this is going. What, if, then do we? Can we regulate that, can we say, as could we write a human law that said, every AI system above a level three computational system has to have an amygdala, misery reducing algorithm inside of it, and it must be adequate, and we define that in some concrete way or have some board of AI ethicists who get to kind of reject or allow kind of an FDA.
Speaker 1:Do we need, like an FDA of AIs, that kind of?
Speaker 3:yeah, the FDA AI, the FDA AI.
Speaker 1:The Food and Drug Administration Intelligence Agency.
Speaker 2:I think, yeah, I think it's again, it's too nebulous. Like I think your loan application network model that will approve or disprove your loan application is going to have a certain amount of testing and accuracy and demographic stuff and all the ethics all the ethics should have already happened right. So I think we're not talking about that. We're talking about more generalized things and I think it depends on the context of the other thing and sometimes like the easiest thing to do. Again, is this like preemptive, like should we be doing this as well? As then, if that's a yes, you know in operation or after the fact, then is there a way that we need to be monitoring this?
Speaker 2:And I think the I mean I can't I haven't read any of the other proposals, but the European Union AI law that has been rewritten but it's coming in soon is going to be it's very much like contextual, like are you using this type of technology which has this kind of capability over here? And if so, you have to tell us. You literally have to tell us and we're going to, we're going to look at it. So there's, there's ways of like operating right, but then everyone says it's stifling innovation, this, you know this. Look at the pharmaceutical industry.
Speaker 1:It's a huge industry that makes billions and billions of dollars and they spend on average, a billion dollars to get a drug to market and a big chunk of that is just testing that the FDA requires. And they still do it and they make money and they make new drugs. Every year, new drugs come out. So if these AIs are going to replace all taxi drivers, that's billions and billions and billions of dollars, billions of dollars of money way more than like a new, a new antidepressant drug. So like why can't we have some, you know, some like requirements, maybe like phases, like the FDA?
Speaker 2:You go to phase one, phase two trials. Why don't we?
Speaker 1:I would, I would, I think that's. I mean I don't love, I don't have a love in my heart for the FDA, but but I think that you know it's. It seems like it's not a terrible thing to think about in terms of having for AI.
Speaker 2:Yeah, yeah, yeah, definitely. I think that the thing is that where the lines are at the moment, like because AI is just like a bunch of like algorithms, essentially it's like actually just letting people know like you're a startup, you're making this thing and it's using this kind of data and you're using these types of algorithms and it's going to be applied to this area and it could affect, you know, a million people. Let's say, like those are, those are things that you can say and then we can, you know, quantifiably say okay, you need to tell us all this stuff or you need to come in and show us, or whatever it is, and this is slightly more easy to police.
Speaker 1:So if you come in and say I'm going to make an AI that fires guns based on visual? You know, then it's like you need to come in like right now.
Speaker 2:Right now, we're going to really review this. But if you say yeah, we're going to make an.
Speaker 1:AI. That helps, you know, helps like people write college essays. It's like, well, yeah, whatever you know, do whatever you want, yeah yeah, I would suggest that that's probably a problematic area as well.
Speaker 2:But, like the, the counter argument as well is that because they should be writing their own essays, you mean they should be writing their own essays. Yeah, Um is because this is a technology that people can just use. So, unlike you, know biotech, it's very difficult to have a bio lab in your house, whereas we all have computers in our houses.
Speaker 1:You know, it says right right right. And you can use compute computers up in the cloud and you can just access it through Amazon computers and you can pay for computers in the cloud and you could probably buy data.
Speaker 2:So it's a slightly different problem because of that, those things, and that we have to police it in a way that actually makes sense for not business, like, the thing that worries me the most is not business because they have to operate in the public, right, it's everything else.
Speaker 1:It's rogue rogue operators.
Speaker 2:Yeah, it's just people who have like Stanley or Stanley or wait who's the who's the unit bombers name Ted Kuzinski.
Speaker 1:Yeah, ted Kuzinski of AI. It could be a dangerous thing.
Speaker 2:Yeah.
Speaker 3:Yeah, yeah. Should I be ashamed that I just had that right ready to go? Scott's, like in his bunker, like I don't remember who this guy is I have up on the wall right here.
Speaker 1:Ted Kuzinski actually had a very interesting critique of technology. I mean not that we should listen to total psychos, but you know he did have a manifesto about technology ruining society and that was part of what drove him to do the bombing that he did so some people are saying it's actually having a revival. Ted Kuzinski's manifesto is being read by zoomers, by younger generation.
Speaker 3:Cool, I'm sure that'll go really well.
Speaker 1:Well, because there's a pushback against all this technology. Well, okay.
Speaker 3:So here's the part where I have to ask do you want people to contact you, and how would be the best ways for them to do it?
Speaker 2:Yes, I'd love that. So you can go to Ben by foodcom. You can check out the podcast machine dash ethicsnet and you can find me on LinkedIn, Ben by foods. And yeah, talk to me, I am me and if you are in this world then I will interview you.
Speaker 3:And it'll be cool and that's been the way you would spell, but by for it is B Y F O R D and we'll put it on the link in the links in the notes.
Speaker 1:Yeah, this has been so fascinating, ben, you can tell I've been like I just have more and more talking because you know we did a one episode and just more to say but so exciting and interesting. Thanks so much for your expertise and time Also just for listeners.
Speaker 3:I just went to Ben by foodcom and you have a fantastically interactive. Oh, it's so cool.
Speaker 2:Yeah, it's really cool, but I can't describe you move your cursor around, you get a pixelation process.
Speaker 3:That is yeah To me, so check it out for that reason, if no other, but check it out for all the other reasons as well, of course.
Speaker 2:Thank you for having me, guys.
Speaker 3:Thanks for being here, Ben. It's great meeting you Take care, all right, bye.
Speaker 2:Yeah, oh, I really need a wheel.